The role of machine learning in climate change research
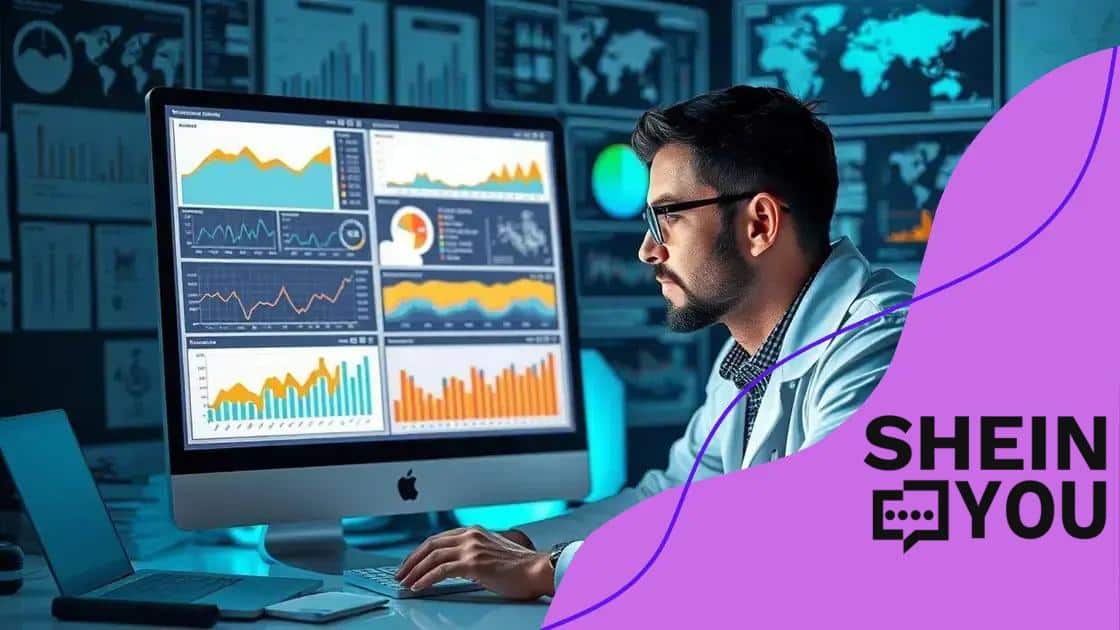
The role of machine learning in climate change research includes improving predictive accuracy, analyzing vast datasets, and enabling innovative solutions for sustainability and resource management.
The role of machine learning in climate change research is becoming increasingly vital as we seek effective solutions to this global crisis. Have you ever wondered how technology can help us understand complex climate data? Let’s dive in!
Understanding machine learning basics
Understanding machine learning basics is essential for grasping how we apply technology to tackle climate change. Machine learning (ML) is a branch of artificial intelligence that enables computers to learn from data. This learning process can yield insights that are vital for climate research.
ML algorithms analyze vast amounts of data to identify patterns and trends. One example is using historical climate data to predict future weather patterns. This data-driven approach helps researchers make informed decisions. To dive deeper into ML, it’s useful to know its key concepts.
Key concepts of machine learning
Understanding some foundational concepts will enhance your grasp of ML:
- Algorithms: These are the mathematical procedures that process data. Different algorithms can yield different insights based on the same data set.
- Training: This refers to the process of teaching the algorithm using labeled data. It’s like teaching a child by examples.
- Testing: After training, the algorithm needs evaluation with different data to ensure it learns correctly.
- Model: The final output after training is called a model, which can make predictions on new data.
As researchers use ML, they can refine models for specific climate-related tasks. For instance, they might develop models to predict greenhouse gas emissions or to analyze satellite imagery for deforestation patterns. This adaptability makes ML a powerful tool in climate change research.
Additionally, the importance of collaboration cannot be overstated. Climate scientists often work together with data scientists to enhance their modeling accuracy. Each team brings unique insights that can improve outcomes in climate predictions. Working in this interdisciplinary manner helps leverage the strengths of both fields.
Machine learning not only helps in analyzing climate data but also offers solutions to mitigate adverse effects. Technologies that utilize ML can optimize energy usage or improve resource allocation during natural disasters. This potential is crucial for creating sustainable solutions for our planet.
Applications of machine learning in climate models
Applications of machine learning in climate models offer exciting opportunities to enhance our understanding of climate change. By integrating machine learning techniques into climate models, scientists can analyze complex data sets more efficiently and accurately, leading to better predictions.
One vital application is in improving weather forecasting. Machine learning algorithms can process vast amounts of meteorological data to identify patterns that traditional models might miss. This ensures that we receive timely and accurate forecasts, which are crucial for disaster preparedness.
Predicting climate outcomes
Additionally, machine learning can predict long-term climate outcomes. By using historical climate data along with current variables, algorithms can model different scenarios. These predictions help policymakers make informed decisions. Here are some ways ML contributes:
- Trend analysis: ML identifies trends in temperature changes over decades.
- Impact assessment: It evaluates how climate change affects various ecosystems.
- Renewable energy optimization: ML optimizes energy output from renewable sources by predicting demand patterns.
Another significant area is in data assimilation. Machine learning can integrate real-time data from various sources, like satellites and ground sensors. This process enhances model accuracy and ensures researchers are working with the most relevant information.
The adaptability of machine learning is another crucial aspect. As models evolve, ML can learn from new data continually, improving predictions over time. For example, analyzing sea level rise projections or carbon footprint reductions becomes more accurate with each model iteration. This leads to more reliable insights for scientists and policymakers alike.
Moreover, machine learning plays a vital role in climate simulations. By using algorithms to run simulations faster, researchers can assess the impacts of various climate interventions, such as different carbon emission scenarios. The result is a more comprehensive understanding of potential climate futures, which can guide strategies for mitigation.
Data analysis and prediction accuracy
Data analysis and prediction accuracy are critical components of utilizing machine learning in climate change research. Effective data analysis helps scientists understand climate trends, while prediction accuracy ensures that policies are based on reliable forecasts.
Machine learning enhances data analysis by allowing for the processing of large and complex datasets. This data can come from various sources, like satellites, weather stations, and ocean buoys. As a result, researchers can obtain a comprehensive picture of climate patterns. One important aspect of this process is data cleaning, where errors or inconsistencies in the data are corrected.
Techniques for improving prediction accuracy
Several techniques can be employed to improve the accuracy of predictions. For example:
- Cross-validation: This method evaluates how the conclusions of a statistical analysis will generalize to an independent data set.
- Feature selection: This identifies the most relevant variables that influence the outcomes, making models simpler and more effective.
- Ensemble methods: These involve combining multiple models to produce better predictive performance.
By applying these techniques, scientists can increase the reliability of their climate models. Machine learning algorithms can adjust based on feedback from prediction outcomes, refining their accuracy over time. For instance, if a model predicts temperature changes but does not align with actual measurements, it can learn and adjust accordingly.
Another dimension of improving prediction accuracy involves utilizing real-time data. By incorporating real-time inputs into models, researchers can better predict sudden climate phenomena, like heatwaves and severe storms. This ability to harness timely data leads to actionable insights that can save lives and mitigate damage.
Furthermore, collaboration among climate scientists and data analysts plays a vital role in data analysis and prediction accuracy. These professionals work together to ensure that models consider various factors affecting climate change. Sharing expertise leads to improvements in methodologies and enhances the reliability of predictions.
Case studies of successful climate projects
Case studies of successful climate projects showcase how machine learning contributes to effective climate solutions. By analyzing real-world examples, we can understand the impact of technology on sustainability.
One notable project is the use of machine learning to optimize energy consumption in cities. This project involved collecting data from smart meters to analyze electricity usage patterns. By applying machine learning algorithms, city planners could identify peak usage times and implement strategies to reduce energy costs and emissions.
Examples of successful applications
Here are some successful climate projects utilizing machine learning:
- Google’s Project Sunroof: This initiative uses machine learning to analyze satellite imagery, helping homeowners determine the solar potential of their roofs. By leveraging this data, more people can invest in solar energy.
- The Climate Corporation: This company uses machine learning to provide farmers with insights on crop yields and weather patterns. Their technology helps farmers make better decisions for sustainable farming practices.
- Wildlife conservation efforts: Machine learning is employed to monitor endangered species. Algorithms analyze images from camera traps, allowing conservationists to track populations and behaviors effectively.
In addition to these examples, many cities are leveraging machine learning to improve waste management. By analyzing waste collection patterns, cities can optimize routes and reduce greenhouse gas emissions. This not only enhances operational efficiency but also lowers costs.
Machine learning is instrumental in predicting climate events. For instance, certain projects focus on analyzing ocean data to predict severe weather patterns like hurricanes. By accurately forecasting these events, communities can prepare and respond more effectively. This proactive approach saves both lives and resources.
Learning from successful projects can inspire further innovations. As machine learning becomes more integrated into climate action, it promises to provide valuable tools for researchers, policymakers, and communities across the globe.
Future trends in machine learning for climate research
Future trends in machine learning for climate research are shaping how scientists will address climate change. As technology continues to advance, machine learning techniques are becoming increasingly sophisticated, leading to new possibilities in climate studies.
One significant trend is the integration of big data with machine learning. As we collect more data from sensors, satellites, and other sources, algorithms need to process this information efficiently. Enhanced computational power will allow machine learning models to analyze vast datasets faster and more accurately.
Emerging technologies in climate research
Several emerging technologies will impact machine learning’s role in climate research:
- Edge computing: This technology processes data at the source, reducing latency and improving response times. It will be especially useful in remote areas for monitoring environmental changes.
- Generative adversarial networks (GANs): GANs are powerful algorithms capable of generating realistic simulation data. This will help scientists create better climate models and visualize different scenarios.
- Cloud computing: Access to cloud resources allows researchers to collaborate globally and share large datasets. This will enhance the training of machine learning models across various institutions.
Another critical trend is the focus on interpretability in machine learning. As climate models become more complex, understanding how they arrive at certain predictions is crucial. Researchers are developing techniques to make machine learning models more transparent and trustworthy. This ensures that policymakers can make informed decisions based on the predictions provided.
Collaboration between climate scientists and data scientists will continue to grow. As both fields merge, innovative solutions for climate adaptation and mitigation will emerge. Projects that utilize machine learning will increasingly focus on real-world applications, such as improving resource efficiency and reducing emissions.
Additionally, ethical considerations will gain prominence. As machine learning influences policy decisions, ensuring fair and responsible use of technology will be vital. Researchers will need to address questions regarding data privacy, bias in algorithms, and how best to communicate findings to the public.
FAQ – Frequently Asked Questions about Machine Learning in Climate Change Research
How does machine learning improve climate predictions?
Machine learning analyzes large datasets to identify patterns, resulting in more accurate climate predictions and better insights for decision-making.
What are some examples of machine learning applications in climate research?
Examples include optimizing energy consumption, predicting weather patterns, and enhancing wildlife conservation efforts.
Why is data analysis important in climate research?
Data analysis helps researchers understand complex climate data, leading to more effective strategies for combating climate change.
What role will future technologies play in climate research?
Emerging technologies like big data, cloud computing, and ethical AI practices will drive innovation and effectiveness in climate solutions.